
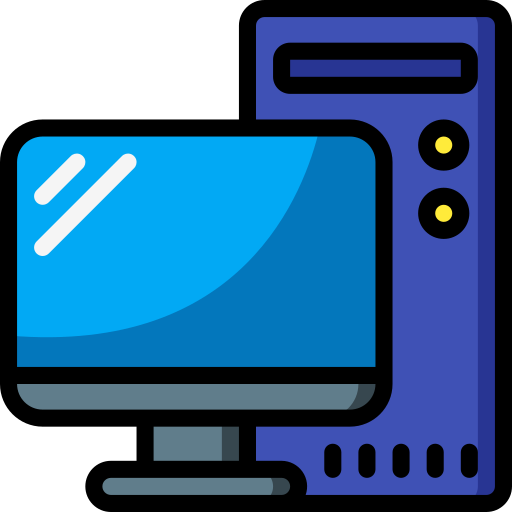
Seems the chapter for Jellyfin has been “coming soon” for 3 years, too bad.
Just a stranger trying things.
Seems the chapter for Jellyfin has been “coming soon” for 3 years, too bad.
I’m not saying it’s not true, but nowhere on that page is there the word donation. And if it is, the fact that it is described and a license, tied to a server or a user causes a lot of confusion to me, especially when combined with the fact that there is no paywall but that it requires registration.
Why use the term license, server and user? Why not simply say donation and with the option of displaying the support by getting exclusive access to a badge like signal does?
Again, I’m very happy immich is free, it is great software and it deserves support but this is just super confusing to me and the buy.immich.app link does not clarify things nor does that blog post.
Edit: typo
Hi and thank you so much for the fantastic work on Immich! I’m hoping to get a chance to try it out soon, with the first stable release!
One question on the financial support page: is it not a donation? There is a per server and a per user purchase, but I thought immich was exclusively self hosted, is it not? Or is this more like a way to say thanks while giving some hints as to how immich is being used privately? Or is there a way to actually pay to have immich host a server for one?
Thanks for clarifying!
This is the way.
I hear you, but how much time was Synology given? If it was no time at all (which it seems is what happened here??), that does not even give Synology a chance and that’s what I’m concerned with. If they get a month (give or take), then sure, disclose it and too bad for them if they don’t have a fix, they should have taken it more seriously, but I’m wondering about how much time they were even given in this case.
Was it that the talk was a last minute change (replacing another scheduled talk) so the responsible disclosure was made in a rush without giving synology more time to provide the patch before the talk was presented?
If so, who decided it was a good idea to present something regarding a vulnerability without the fix being available yet?
I’m not sure, I read that ZFS can help in the case of ransomware, so I assumed it would extend to accidental formatting but maybe there’s a key difference.
I think these kind of situations are where ZFS snapshots shine: you’re back in a matter of seconds with no data loss (assuming you have a recent snapshot before the mistake).
Edit: yeah no, if you operate at the disk level directly, no local ZFS snapshot could save you…
I didn’t say it can’t. But I’m not sure how well it is optimized for it. From my initial testing it queues queries and submits them one after another to the model, I have not seen it batch compute the queries, but maybe it’s a setup thing on my side. vLLM on the other hand is designed specifically for the multi co current user use case and has multiple optimizations for it.
I run the Mistral-Nemo(12B) and Mistral-Small (22B) on my GPU and they are pretty code. As others have said, the GPU memory is one of the most limiting factors. 8B models are decent, 15-25B models are good and 70B+ models are excellent (solely based on my own experience). Go for q4_K models, as they will run many times faster than higher quantization with little performance degradation. They typically come in S (Small), M (Medium) and (Large) and take the largest which fits in your GPU memory. If you go below q4, you may see more severe and noticeable performance degradation.
If you need to serve only one user at the time, ollama +Webui works great. If you need multiple users at the same time, check out vLLM.
Edit: I’m simplifying it very much, but hopefully should it is simple and actionable as a starting point. I’ve also seen great stuff from Gemma2-27B
Edit2: added links
Edit3: a decent GPU regarding bang for buck IMO is the RTX 3060 with 12GB. It may be available on the used market for a decent price and offers a good amount of VRAM and GPU performance for the cost. I would like to propose AMD GPUs as they offer much more GPU mem for their price but they are not all as supported with ROCm and I’m not sure about the compatibility for these tools, so perhaps others can chime in.
Edit4: you can also use openwebui with vscode with the continue.dev extension such that you can have a copilot type LLM in your editor.
Indeed, quite surprising. You got to “stroke their fur the right way” so to speak haha
Also, I’m increasingly more impressed with the rapid progress reaching open-weights models: initially I was playing with Llama3.1-8B which is already quite useful for simple querries. Then lately I’ve been trying out Mistral-Nemo (12B) and Mistrall-Small (22B) and they are quite much more capable. I have a 12GB GPU and so far those are the most powerful models I can run decently. I’m using them to help me in writing tasks for ansible, learning the inner workings of the Linux kernel and some bootloader stuff. I find them quite helpful!
Someone recently referred me to this blog post about using RAG in open-webui. I have not tested if but the author seems to reach a good setup.
Perhaps this is of use to you?
I have no idea if ollama can handle multi-GPU. The 70B in it’s q2_k quantized form requires already 26GB of memory, so you would need at least that to run it well and that would only imply it could be entirely run on GPU, which is the best case scenario, but not at what speed.
I know some people with apple silicon who have enough memory to run the 70B model and for them it runs fast enough to be usable. You may be able to find more info about it online.
I wish I could. I have an RTX 3060 12GB, I run mostly llama3.1 8B versions in fp8, at 30-35 tokens/s.
Sure! It can be a bit of a steep learning curve at times but there are heaps of resources online, and LLMs can also be useful, even if it just in pointing you in the direction for further reading. Regardless, you can reach out to me or other great folks from the !localllama@sh.itjust.works or similar AI, ML or related communities!
Enjoy :)
For RAG, there are some tools available in open-webui, which are documented here: https://docs.openwebui.com/tutorials/features/rag They have plans for how to expand and improve it, which they describe here: https://docs.openwebui.com/roadmap#information-retrieval-rag-
For fine-tuning, I think this is (at least for now) out of scope. They focus on inferencing. I think the direction is to eventually help you create/manage your own data which you get from using LLMs using Open-WebUI, but the task of actually fine-tuning is not possible (yet) using either ollama or open-webui.
I have not used the RAG function yet, but besides following the instructions on how to set it up, your experience with RAG may also be somewhat limited depending on which embedding model you use. You may have to go and look for a good model (which is probably both small and efficient to re-scan your documents yet powerful to generate meaningful embeddings). Also, in case you didn’t know, the embeddings you generate are specific to an embedding model, so if you change that model you’ll have to rescan your whole documents library.
Edit: RAG seems a bit limited by the supported file types. You can get it here: https://github.com/open-webui/open-webui/blob/2fa94956f4e500bf5c42263124c758d8613ee05e/backend/apps/rag/main.py#L328 It seems not to support word documents, or PDFs, so mostly incompatible with documents which have advanced formatting and are WYSIWYG.
The interface called open-webui can run in a container, but ollama runs as a service on your system, from my understanding.
The models are local and only answer queries by default. It all happens on the system without any additional tools. Now, if you want to give them internet access, you can, it is an option you have to setup and open-webui makes that possible though I have not tried it myself. I just see it.
I have never heard of any llm “answer base queries offline before contacting their provider for support”. It’s almost impossible for the LLM to do it by itself without you setting things up for it that way.
whats great is that with ollama and webui, you can as easily run it all on one computer locally using the open-webui pip package or in a remote server using the container version of open-webui.
Ive run both and the webui is really well done. It offers a number of advanced options, like the system prompt but also memory features, documents for RAG and even a built in python ide for when you want to execute python functions. You can even enable web browsing for your model.
I’m personally very pleased with open-webui and ollama and they both work wonders together. Hoghly recommend it! And the latest llama3.1 (in 8 and 70B variants) and llama3.2 (in 1 and 3B variants) work very well, even on CPU only, for the latter! Give it a shot, it is so easy to set up :)
This is great! I had wondered, with my GoG games, as they provide offline installers for the games, what would be the best way to manage and distribute them for myself and this really hits the spot! Very glad to be able to folly self-manage my games in this way. Thanks!
Ollama is very useful but also rather barebones. I recommend installing Open-Webui to manage models and conversations. It will also be useful if you want to tweak more advanced settings like system prompts, seed, temperature and others.
You can install open-webui using docker or just pip, which is enough if you only care about serving yourself.
Edit: open-webui also renders markdown, which makes formatting and reading much more appealing and useful.
Edit2: you can also plug ollama into continue.dev, an extension to vscode which brings the LLM capabilities to your IDE.