
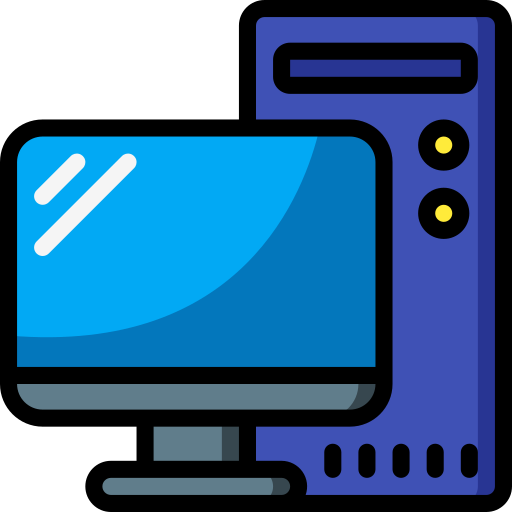
No, all sizes of llama 3.1 should be able to handle the same size context. The difference would be in the “smarts” of the model. Bigger models are better at reading between the lines and higher level understanding and reasoning.
No, all sizes of llama 3.1 should be able to handle the same size context. The difference would be in the “smarts” of the model. Bigger models are better at reading between the lines and higher level understanding and reasoning.
Wow, that’s an old model. Great that it works for you, but have you tried some more modern ones? They’re generally considered a lot more capable at the same size
Increase context length, probably enable flash attention in ollama too. Llama3.1 support up to 128k context length, for example. That’s in tokens and a token is on average a bit under 4 letters.
Note that higher context length requires more ram and it’s slower, so you ideally want to find a sweet spot for your use and hardware. Flash attention makes this more efficient
Oh, and the model needs to have been trained at larger contexts, otherwise it tends to handle it poorly. So you should check what max length the model you want to use was trained to handle
I still use http a lot for internal stuff running in my own network. There’s no spying there… I hope … And ssl for local network only services is a total pita.
So I really hope browsers won’t adapt https only
But even if you use GoMommy extra super duper triple snake oil security checked ssl cert, if I trick LetsEncrypt to sign a key for that domain I still have a valid cert for your site.
I doubt the disk will bottleneck at 40mb/s when doing sequential write. Torrent downloads are usually heavy random writes, which is the worst you can do to a HDD.
Llama3 8b can be run at 6gb vram, and it’s fairly competent. Gemma has a 9b I think, which would also be worth looking into.
Better background backups
Rework background backups to be more reliable
Hilarious for a system which main point / feature is photo backup
It’s less the calculations and more about memory bandwidth. To generate a token you need to go through all the model data, and that’s usually many many gigabytes. So the time it takes to read through in memory is usually longer than the compute time. GPUs have gb’s of RAM that’s many times faster than the CPU’s ram, which is the main reason it’s faster for llm’s.
Most tpu’s don’t have much ram, and especially cheap ones.
Reasonable smart… that works preferably be a 70b model, but maybe phi3-14b or llama3 8b could work. They’re rather impressive for their size.
For just the model, if one of the small ones work, you probably need 6+ gb VRAM. If 70b you need roughly 40gb.
And then for the context. Most models are optimized for around 4k to 8k tokens. One word is roughly 3-4 tokens. The VRAM needed for the context varies a bit, but is not trivial. For 4k I’d say right half a gig to a gig of VRAM.
As you go higher context size the VRAM requirement for that start to eclipse the model VRAM cost, and you will need specialized models to handle that big context without going off the rails.
So no, you’re not loading all the notes directly, and you won’t have a smart model.
For your hardware and use case… try phi3-mini with a RAG system as a start.
For the nfs shares, there’s generally two approaches to that. First is to mount it on host OS, then map it in to the container. Let’s say the host has the nfs share at /nfs, and the folders you need are at /nfs/homes. You could do “docker run -v /nfs/homes:/homes smtpserverimage” and then those would be available from /homes inside the image.
The second approach is to set up NFS inside the image, and have that connect directly to the nfs server. This is generally seen as a bad idea since it complicates the image and tightly couples the image to a specific configuration. But there are of course exceptions to each rule, so it’s good to keep in mind.
With database servers, you’d have that set up for accepting network connections, and then just give the address and login details in config.
And having a special setup… How special are we talking? If it’s configuration, then that’s handled by env vars and mapping in config files. If it’s specific plugins or compile options… Most built images tend to cast a wide net, and usually have a very “everything included” approach, and instructions / mechanics for adding plugins to the image.
If you can’t find what you’re looking for, you can build your own image. Generally that’s done by basing your Dockerfile on an official image for that software, then do your changes. We can again take the “postgres” image since that’s a fairly well made one that has exactly the easy function for this we’re looking for.
If you would like to do additional initialization in an image derived from this one, add one or more *.sql, *.sql.gz, or *.sh scripts under /docker-entrypoint-initdb.d (creating the directory if necessary). After the entrypoint calls initdb to create the default postgres user and database, it will run any *.sql files, run any executable *.sh scripts, and source any non-executable *.sh scripts found in that directory to do further initialization before starting the service.
So if you have a .sh script that does some extra stuff before the DB starts up, let’s say “mymagicpostgresthings.sh” and you want an image that includes that, based on Postgresql 16, you could make this Dockerfile in the same folder as that file:
FROM postgres:16
RUN mkdir /docker-entrypoint-initdb.d
COPY mymagicpostgresthings.sh /docker-entrypoint-initdb.d/mymagicpostgresthings.sh
RUN chmod a+x /docker-entrypoint-initdb.d/mymagicpostgresthings.sh
and when you run “docker build . -t mymagicpostgres” in that folder, it will build that image with your file included, and call it “mymagicpostgres” - which you can run by doing “docker run -e POSTGRES_PASSWORD=mysecretpassword -p 5432:5432 mymagicpostgres”
In some cases you need a more complex approach. For example I have an nginx streaming server - which needs extra patches. I found this repository for just that, and if you look at it’s Dockerfile you can see each step it’s doing. I needed a bit of modifications to that, so I have my own copy with different nginx.conf, an extra patch it downloads and applies to the src code, and a startup script that changes some settings from env vars, but that had 90% of the work done.
So depending on how big changes you need, you might have to recreate from scratch or you can piggyback on what’s already made. And for “docker script to launch it” that’s usually a docker-compose.yml file. Here’s a postgres example:
version: '3.1'
services:
db:
image: postgres
restart: always
environment:
POSTGRES_PASSWORD: example
adminer:
image: adminer
restart: always
ports:
- 8080:8080
If you run “docker compose up -d” in that file’s folder it will cause docker to download and start up the images for postgres and adminer, and port forward in 8080 to adminer. From adminer’s point of view, the postgres server is available as “db”. And since both have “restart: always” if one of them crashes or the machine reboots, docker will start them up again. So that will continue running until you run “docker compose down” or something catastrophic happens.
It’s a great tool to have in the toolbox. Might take some time to wrap your head around, but coming from vm’s you already have most of the base understanding.
From a VM user’s perspective, some translations:
A small tip: you can “exec” into a running container, which will run a command inside that container. Combined with interactive (-i) and terminal (-t) flags, it’s a good way to get a shell into a running container and have a look around or poke things. Sort of like getting a shell on a VM.
One thing that’s often confusing for new people are image tags. Partially because it can mean two things. For example “postgres” is a tag. That is attached to an image. The actual “name” of an image is it’s Sha sum. An image can have multiple tags attached. So far so good, right?
Now, let’s get complicated. The actual tag, the full tag for “postgres” is actually “docker.io/postgres:latest”. You see, every tag is a URL, and if it doesn’t have a domain name, docker uses it’s own. And then we get to the “: latest” part. Which is called a tag. Yup. All tags have a tag. If one isn’t given, it’s automatically set to “latest”. This is used for versioning and different builds.
For example postgres have tags like “16.1” which points to latest 16.1.x version image, built on postgres maintainers’ preferred distro. “16.1-alpine” that point to latest Alpine based 16.1.x version. “16” for latest 16.x.x version, “alpine” for latest alpine based version, be it 16 or 17 or 18… and so on. You can find more details here.
The images on docker hub are made by … well, other people. Often the developers of that software themselves, sometimes by docker, sometimes by random people. You can make your own account there, it’s free. If you do, make an image and pushes it, it will be available as shdwdrgn/name - if it doesn’t have a user component it’s maintained / sanctioned by docker.
You can also run your own image repository service, as long as it has https with valid cert. Then it will be yourdomain.tld/something
So that was a brief introduction to the strange World of docker. Docker is a for profit company, btw. But the image format is standardized, and there’s fully open source ways to make and run images too. At the top of my head, podman and Kubernetes.
Yeah, it also has the effect that when starting up say a new postgres or web server is one simple command, a few seconds and a few mb of disk and ram, you do it more for smaller stuff.
Instead of setting up one nginx for multiple sites you run one nginx per site and have the settings for that as part of the site repository. Or when a service needs a DB, just start a new one just for that. And if that file analyzer ran in it’s own image instead of being part of the web service, you could scale that separately… oh, and it needs a redis instance and a rabbitmq server, that’s two more containers, that serves just that web service. And so on…
Things that were a huge hassle before, like separate mini vm’s for each sub-service, and unique sub-services for each service doesn’t just become practical but easy. You can define all the services and their relations in one file and docker will recreate the whole stack with all services with one command.
And then it also gets super easy to start more than one of them, for example for testing or if you have a different client. … which is how you easily reach a hundred instances running.
So instead of a service you have a service blueprint, which can be used in service stack blueprints, which allows you to set up complex systems relatively easily. With a granularity that would traditionally be insanity for anything other than huge, serious big-company deployments.
Nine. How much ram do they use? How much disk space? Try running 90, or 900. Currently, on my personal hobby kubernetes cluster, there’s 83 different instances running. Because of the low overhead, I can run even small tools in their own container, completely separate from the rest. If I run say… a postgresql server… spinning one up takes 90mb disk space for the image, and about 15 mb ram.
I worked at a company that did - among other things - hosting, and was using VM’s for easier management and separation between customers. I wasn’t directly involved in that part day to day, but was friend with the main guy there. It was tough to manage. He was experimenting with automatic creating and setting up new VM’s, stripping them for unused services and files, and having different sub-scripts for different services. This was way before docker, but already then admins were looking in that direction.
So aschually, docker is kinda made for people who runs things in VM’s, because that is exactly what they were looking for and duct taping things together for before docker came along.
Just remember that Raspberry is an ARM cpu, which is a different architecture. Docker can cross compile to it, and make multiple images automatically. It takes more time and space though, as it runs an arm emulator to make them.
https://www.docker.com/blog/faster-multi-platform-builds-dockerfile-cross-compilation-guide/ has some info about it.
VM’s have much bigger overhead, for one. And VM’s are less reproducible too. If you had to set up a VM again, do you have all the steps written down? Every single step? Including that small “oh right” thing you always forget? A Dockerfile is basically just a list of those steps, written in a way a computer can follow. And every time you build an image in docker, it just plays that list and gives you the resulting file system ready to run.
It’s incredibly practical in some cases, let’s say you want to try a different library or upgrade a component to a newer version. With VM’s you could do it live, but you risk not being able to go back. You could make a copy or make a checkpoint, but that’s rather resource intensive. With docker you just change the Dockerfile slightly and build a new image.
The resulting image is also immutable, which means that if you restart the docker container, it’s like reverting to first VM checkpoint after finished install, throwing out any cruft that have gathered. You can exempt specific file and folders from this, if needed. So every cruft and change that have happened gets thrown out except the data folder(s) for the program.
Modularity, compartmentalization, reliability, predictability.
One software needs MySQL 5, another needs mariadb 7. A third service needs PHP 7 while the distro supported version is 8. A fourth service uses cuda 11.7 - not 11.8 which is what everything in your package manager uses. a fifth service’s install was only tested on latest Ubuntu, and now you need to figure out what rpm gives the exact library it expects. A sixth service expects odbc to be set up in a very specific way, but handwaves it in the installation docs. A seventh program expects a symlink at a specific place that is on the desktop version of the distro, but not the server version. And then you got that weird program that insist on admin access to the database so it can create it’s own user. Since I don’t trust it with that, let it just have it’s own database server running in docker and good riddance.
And so on and so forth… with docker not only is all this specified in excruciating details, it’s also the exact same setup on every install.
You don’t have it not working on arch because the maintainer of a library there decided to inline a patch that supposedly doesn’t change anything, but somehow causes the program to segfault.
I can develop a service on windows, test it, deploy it to my Kubernetes cluster, and I don’t even have to worry about which machine to deploy it on, it just runs it on a machine. Probably an Ubuntu machine, but maybe on that Gentoo node instead. And if my osx friend wants to try it out, then no problem. I can just give him a command, and it’s running on his laptop. No worries about the right runtime or setting up environment or libraries and all that.
If you’re an old Linux admin… This is what utopia looks like.
Edit: And restarting a container is almost like reinstalling the OS and the program. Since the image is static, restarting the container removes all file system cruft too and starts up a pristine new copy (of course except the specific files and folders you have chosen to save between restarts)
Had a router / firewall salesman talk about a firewall’s ddos protection once. I kinda laughed in his face and asked how they did that feat.
Of course, he had no clue. But was still very confident in it. After all, it said so on the box.
Probably running a local model, like for example Mistral. Sounds like small local model + RAG
And then it doesn’t work on one VM and it takes you three days to figure out that VM had a slightly different version of a library and that makes the app segfault.