
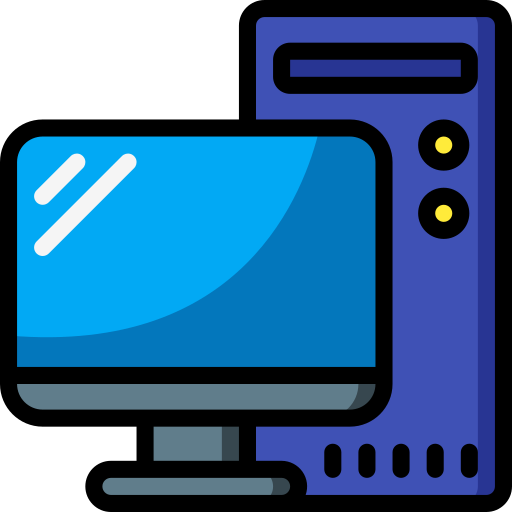
You can drploy a Cloudflare worker that exposes an APi endpoint with an SQLite DB completely for free and without doing any maintenance. I don’t think the DB is encrypted , so it wouldn’t be my first choice if privacy is a concern. There’s a bit of a learning curve with all the UI bloat but once you figured it out it’s a very hassle free solution.
This is honestly awesome! I was thinking about a similar setup for a long time but wasn’t sure how to do this exactly, this seems exactly like the setup I was looking for. Thank you!